What is Data Cleaning and Why Does It Matter?
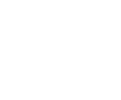
When working with data, many believe that the quality of your findings and interpretations directly reflects the quality of the data you utilize. But, if you input poor data, you’ll get poor results. Before delving into the intricacies of data cleaning, it’s pivotal to note that verification and reporting come directly before the data-cleaning process. Data cleaning, sometimes called data cleansing or scrubbing, is crucial for your organization. So, what is data cleaning? It’s a vital step if you aim to foster a culture centered on making decisions based on top-notch data.
Data cleaning involves refining, adjusting, and organizing data within a dataset to make it consistent and ready for examination. This means eliminating flawed or unrelated data and converting it into a format that machine can process efficiently. The data cleaning process steps involve ensuring accuracy, consistency, and completeness in your dataset.
A common phrase in data analysis is: “Garbage in, garbage out.” This implies that starting with poor-quality data will yield better-quality outcomes.
While data cleaning can be time-consuming, it’s crucial for deriving meaningful insights from your data. Before diving into the verification and reporting, it’s essential to explain the data-cleaning process thoroughly.
Data Cleaning
Consider the 1-10-100 principle: Spending $1 to prevent poor data saves $10 in correction costs and $100 in addressing issues caused by insufficient data.
Therefore, thorough data cleaning is vital to achieve the best outcomes.
In machine learning, many data experts believe that high-quality data trumps even the most advanced algorithms. Machine learning models are only as good as the data they learn from.
Training models on flawed data means the final analysis won’t just be unreliable; it could be detrimental to your business.
Effective data cleaning not only conserves resources but also enhances your organization’s productivity, enables precise targeting of specific markets, and permits the reuse of datasets for various analyses and applications.
The Importance of Data Cleaning in the Business
Data cleaning is a crucial part of analyzing data. When done right, it guarantees that the data is accurate, consistent, and complete. This step is vital for organizations aiming for precise and informed choices. Here’s why data cleaning holds significance:
Through the data cleaning process, mistakes, inconsistencies, and wrong information are eliminated, leading to a trustworthy dataset. This guarantees dependable conclusions from the data.
Clean and trustworthy data is the backbone of successful market research. With cleaned data, organizations can trust their analysis and make informed choices based on the data’s insights.
Cleaning data helps in removing repeated entries, fixing typos, and making data formats uniform. After data cleaning, the data is more uniform and user-friendly than its original state.
Data cleaning reduces the time and energy needed to prepare the data for analysis. This lets data experts concentrate on studying the data instead of cleaning it.
When combining data from different places, data cleaning ensures the data remains consistent and trustworthy.
More Data-related articles:
Start by determining the type of analysis you aim for and the questions you want to address. Examine your data closely to identify what’s pertinent and what’s not. Remove any data points that don’t align with your objectives.
For instance, if you’re analyzing SUV owners and your data includes Sedan owners, that could be more useful. It could distort your findings. Also, consider eliminating elements like hashtags, URLs, emojis, and HTML tags unless they’re crucial to your study.
Collecting data from varied sources or departments, using scraped data, or receiving multiple feedback can lead to duplicate data entries.
Duplicates not only consume more storage but also hinder the analysis process. If you use a dataset with repeated entries for machine learning, the model might overemphasize these duplicates. It’s essential to remove them for unbiased outcomes.
Simple data cleaning tools can efficiently identify and remove these duplicates, as they’re straightforward for AI to spot.
Structural issues include typos, inconsistent naming patterns, wrong capitalization, or incorrect terms. While these seem evident, machine learning tools may not catch them, leading to skewed results.
For instance, if one dataset has a ‘women’ column and another has a ‘female’ column, you’d need to unify these titles. Standardizing dates, addresses, and phone numbers is also crucial for clarity.
Data Cleaning Process
Review your data to find missing entries, blank fields, or unanswered queries. These gaps might be due to incomplete data collection or mistakes. Decide if you should discard related data, fill in the gaps manually, or leave them.
Handling missing data will depend on your intended analysis and preprocessing plans. In some cases, reorganizing your data can mitigate the impact of these gaps.
Outliers are data points that differ markedly from others and can impact your evaluation. For instance, if you calculate an average score and one student doesn’t attempt questions, their 0% would drastically affect the average. In such cases, think about excluding this data.
However, a data point being significantly different sometimes means it will affect the final analysis. The presence of an outlier doesn’t necessarily mean it’s irrelevant. Your decision to include or exclude it should be based on the type of analysis and its potential impact.
The last step in data cleaning is to ensure your data is genuine, consistent, and formatted correctly for further processes.
Ask yourself:
Ensure your data is organized and meets your requirements. Cross-reference data points to ensure completeness and accuracy.
Utilize machine learning and AI to ensure your data’s readiness. After a thorough cleaning, you can employ data-wrangling methods and tools for automation.
The integrity and reliability of data are paramount in today’s decision-making processes. As we’ve answered the question “What is data cleaning?” and the process of data cleaning. It is not just a routine task but a critical step that ensures the accuracy, consistency, and usability of data. By investing time and resources into this process, organizations can confidently base their decisions on data accessible from errors and inconsistencies. Remember, in the world of data, quality is king.
If you’re looking for expert assistance in ensuring your data is of the highest quality, don’t hesitate. Contact TECHVIFY today, and let our professionals guide you through the intricacies of data cleaning and other essential data services. Contact TECHVIFY now for unparalleled expertise and support.
Table of ContentsWhat is data cleaning?The Importance of Data Cleaning in the BusinessEnhanced data precisionInformed choicesConsistent data qualityBoosted efficiencySeamless data merging6 Steps of the Data Cleaning ProcessStep 1: Filter out unnecessary dataStep2: Eliminate duplicate dataStep 3: Correct structural inconsistenciesStep 4: Address gaps in dataStep 5: Remove extreme data pointsStep 6: Confirm data accuracyConclusion Technological advancements are paving new paths for companies across different sectors, and the logistics industry is no exception. According to a survey by Gartner, 87% of supply chain professionals plan to invest in enhancing the resilience of their platforms. Logistics encompasses a broad and complex array of…
26 July, 2024
Table of ContentsWhat is data cleaning?The Importance of Data Cleaning in the BusinessEnhanced data precisionInformed choicesConsistent data qualityBoosted efficiencySeamless data merging6 Steps of the Data Cleaning ProcessStep 1: Filter out unnecessary dataStep2: Eliminate duplicate dataStep 3: Correct structural inconsistenciesStep 4: Address gaps in dataStep 5: Remove extreme data pointsStep 6: Confirm data accuracyConclusion The technology sector is advancing at an unprecedented pace, and the HR landscape is evolving right alongside it. To attract top talent, HR professionals and organizations need to stay ahead of emerging technology hiring trends. This year, we are witnessing significant shifts in hiring practices that will…
25 July, 2024
Table of ContentsWhat is data cleaning?The Importance of Data Cleaning in the BusinessEnhanced data precisionInformed choicesConsistent data qualityBoosted efficiencySeamless data merging6 Steps of the Data Cleaning ProcessStep 1: Filter out unnecessary dataStep2: Eliminate duplicate dataStep 3: Correct structural inconsistenciesStep 4: Address gaps in dataStep 5: Remove extreme data pointsStep 6: Confirm data accuracyConclusion Customized software plays a major role in managing various tasks within the telecom industry. It is essential for allocating numbers to subscribers and managing networks through optimized and AI-enabled routing protocols. Additionally, it aids in detecting fraud with intelligent telecom software development solutions and maintaining detailed subscriber…
24 July, 2024
Thank you for your interest in TECHVIFY Software.
Speed-up your projects with high skilled software engineers and developers.
By clicking the Submit button, I confirm that I have read and agree to our Privacy Policy