LlamaIndex: A Beginner’s Guide
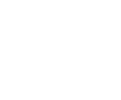
Large Language Models (LLMs) like ChatGPT, crucial for searching and content generation tasks, often need more domain-specific optimization. LlamaIndex addresses this by allowing easy integration of unique data with LLMs, enabling the creation of tailored applications such as enhanced chatbots and knowledge agents. This tool marks a significant advancement in AI, simplifying the development of custom GPT-based applications.
This article will explore the basics of LlamaIndex: definitions, how Llamaindex works, its features and benefits. To help you better understand this new upcoming generative AI framework.
LlamaIndex is a sophisticated framework that boosts the performance of Large Language Models (LLMs) like GPT-4. While LLMs are already powerful due to their training on extensive public datasets, they often need help working with private or specific data types. LlamaIndex solves this problem by providing an organized method to collect, arrange, and use different data sources, such as APIs, databases, and PDFs.
By arranging this data in a way that LLMs can easily use, LlamaIndex enables users to pose queries using everyday language and receive responses from their proprietary data without the need to modify the models. This flexible framework has a simple high-level API for beginners and more detailed options for experts through lower-level APIs. In short, LlamaIndex enhances the power of LLMs, making them more useful and adaptable to specific data needs.
LlamaIndex’s LlamaHub feature is pivotal in its architecture. It allows for ingesting data from a wide range of sources and formats. This flexibility is crucial for businesses and developers dealing with varied data types, enabling them to seamlessly integrate everything from structured databases to unstructured text into the LLM framework.
LlamaIndex supports essential document operations such as inserting, deleting, updating, and refreshing the document index. This functionality ensures that the data remains current and relevant, vital for maintaining the accuracy and reliability of the outputs generated by the LLMs.
One of the standout capabilities of LlamaIndex is its ability to synthesize data from multiple documents or heterogeneous data sources. This feature is particularly beneficial for complex queries that require a comprehensive understanding of various data points, allowing for more nuanced and informed responses.
Including a “Router” feature in LlamaIndex allows users to choose between different query engines. This flexibility is crucial for optimizing query responses based on the application’s specific needs, whether it be speed, accuracy, or a balance of both.
To enhance the quality of its output, LlamaIndex offers hypothetical document embeddings. This advanced feature improves the model’s understanding of the context and nuances within the data, leading to more accurate and relevant responses.
LlamaIndex boasts numerous integrations with various tools and platforms, including vector stores, ChatGPT plugins, tracing tools, LangChain, and more. These integrations extend the functionality of LlamaIndex, allowing it to be used in a wide array of applications and scenarios.
Keeping up with the latest advancements, LlamaIndex supports the latest OpenAI function calling API. This ensures that LlamaIndex remains at the forefront of technology, capable of leveraging the most advanced features offered by OpenAI’s models.
Learn more:
Financial Report Analysis | LlamaIndex can be employed by financial analysts and government agencies to analyze complex financial reports. Integrating with OpenAI can process and interpret data from various fiscal years, aiding in trend analysis, risk assessment, and decision-making in economic sectors. |
Advanced Query Engines | LlamaIndex has been combined with technologies like Ray to develop sophisticated query engines in the tech industry. These engines can handle complex data ingestion and embedding pipelines, making them ideal for businesses that require advanced data retrieval and analysis capabilities. |
Knowledge Agents for Businesses | Companies can leverage LlamaIndex to create intelligent knowledge agents. These agents are trained on specific, custom datasets and highly specialized in certain domains. This application is particularly useful for customer service, market research, and internal knowledge management. |
Academic Research Tools | In the academic field, researchers utilize LlamaIndex to build Retrieval-Augmented Generation (RAG)-based applications. These tools are instrumental in managing and extracting information from extensive collections of research papers and articles, especially in PDF format. This application streamlines the research process, making it easier for scholars to access and synthesize information from many sources. |
Custom Data Integration for AI Applications | Developers use LlamaIndex to integrate various applications’ custom data sources with AI models. This integration enhances the AI’s understanding and response capabilities, making it more relevant and effective in specific contexts. |
Overall, LlamaIndex is revolutionizing the use of AI in various sectors by enhancing Large Language Models like ChatGPT. Its ability to integrate diverse data, manage documents, and synthesize complex information positions it as a vital tool for numerous applications. Whether for financial analysis, academic research, or custom AI applications, LlamaIndex is pivotal in advancing AI capabilities.
Are you looking to leverage the power of AI in your projects? Our team specializes in developing and integrating AI technologies tailored to your needs. Contact TECHVIFY today and transform your business with the latest AI innovation.
Table of ContentsI. Understanding LlamaindexII. How LlamaIndex Works?III. Features and Benefits of Llamaindex01. Data Connectors (LlamaHub)02. Document Operations03. Data Synthesis from Multiple Sources04. Router Feature for Query Engine Selection05. Hypothetical Document Embeddings06. Extensive Integrations07. Support for OpenAI Function Calling APIIV. LlamaIndex Use CasesConclusion Technological advancements are paving new paths for companies across different sectors, and the logistics industry is no exception. According to a survey by Gartner, 87% of supply chain professionals plan to invest in enhancing the resilience of their platforms. Logistics encompasses a broad and complex array of processes that demand the utmost precision and continuous optimization. Companies…
26 July, 2024
Table of ContentsI. Understanding LlamaindexII. How LlamaIndex Works?III. Features and Benefits of Llamaindex01. Data Connectors (LlamaHub)02. Document Operations03. Data Synthesis from Multiple Sources04. Router Feature for Query Engine Selection05. Hypothetical Document Embeddings06. Extensive Integrations07. Support for OpenAI Function Calling APIIV. LlamaIndex Use CasesConclusion The technology sector is advancing at an unprecedented pace, and the HR landscape is evolving right alongside it. To attract top talent, HR professionals and organizations need to stay ahead of emerging technology hiring trends. This year, we are witnessing significant shifts in hiring practices that will redefine our understanding of the future workforce. According to a…
25 July, 2024
Table of ContentsI. Understanding LlamaindexII. How LlamaIndex Works?III. Features and Benefits of Llamaindex01. Data Connectors (LlamaHub)02. Document Operations03. Data Synthesis from Multiple Sources04. Router Feature for Query Engine Selection05. Hypothetical Document Embeddings06. Extensive Integrations07. Support for OpenAI Function Calling APIIV. LlamaIndex Use CasesConclusion Customized software plays a major role in managing various tasks within the telecom industry. It is essential for allocating numbers to subscribers and managing networks through optimized and AI-enabled routing protocols. Additionally, it aids in detecting fraud with intelligent telecom software development solutions and maintaining detailed subscriber profiles, including comprehensive call recording reports. I. A Quick Look…
24 July, 2024
Thank you for your interest in TECHVIFY Software.
Speed-up your projects with high skilled software engineers and developers.
By clicking the Submit button, I confirm that I have read and agree to our Privacy Policy